PROTECT’s Data Management and Analytics Core Receives Supplement to Make Data AI Ready
This September, PROTECT’s Data Management and Analytics Core (DMAC) received supplemental funding from the National Institute of Environmental Health Sciences (NIEHS) to develop a suite of methods to prepare data for large-scale artificial intelligence (AI) and machine learning (ML) analyses. This supplement is part of an NIH initiative to make NIH-supported data suitable for AI/ML analytics.
The project addresses two challenges the PROTECT team faces in its research. The first is missing data, which occurs in virtually all health studies, but has especially impacted the PROTECT team in recent years due to the covid-19 pandemic, Hurricane Maria in 2017, and other major events and systemic issues on the island. Missing data can complicate analyses and may impact research findings.
Some previous analyses used a single imputation approach for missing data, substituting a single value for all missing points in a dataset. Such methods remove any uncertainty from the imputation process and provide no distinction between the original data and new imputations. By contrast, Multiple Imputation (MI) ensures uncertainty is maintained in imputations by completing the same dataset multiple times. While MI is an improvement over single imputation methods, standard MI approaches underperform when relationships among variables are complex. Ensemble methods, like Super Learning (SL), were developed to capture such relationships by optimally combining any number of classical and machine learning models. As such, Multiple imputation by Super Learning (MISL) was developed to generate more efficient and reliable inferences in the presence of complex relationships between observed and missing data. The goal of the research team is to demonstrate the effectiveness of this approach using the PROTECT data set.
The second challenge is the class imbalance inherent in the PROTECT cohort outcomes. The primary aim of the PROTECT Center is to investigate the impacts of environmental contamination on preterm birth outcomes. Because preterm births occur much less frequently than term births, the dataset is imbalanced, with the target outcome—preterm birth—underrepresented. Traditional machine learning methods assume the dataset is balanced, and may interpret the less-common premature births as noise and ignore them. The goal of this project is to develop methods that can mathematically make premature births appear important to these algorithms despite the imbalance.
Addressing both of these challenges will strengthen PROTECT’s analysis capabilities, particularly as researchers begin looking at the complex interactions between mixtures of chemical exposures and their impacts on birth outcomes.
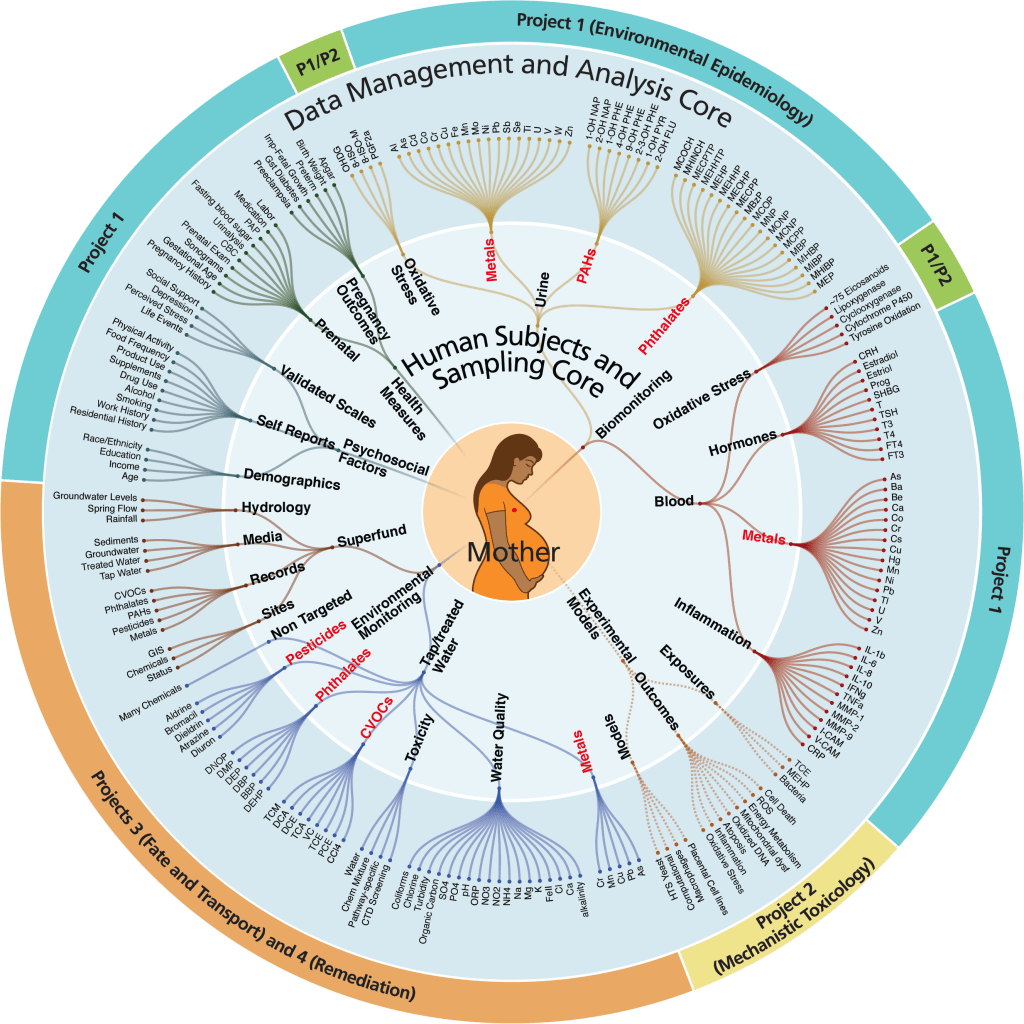
A visualization of all the data variables in the PROTECT database.